$1.19 million grant will leverage single-cell sequencing technology
National Institutes of Health-funded project will help with cancer diagnosis, treatment, prevention
The Texas A&M University System has received a $1.19 million grant from the National Institutes of Health, NIH, for a multidisciplinary collaboration to study the intricate connections between genomics, nutrition and health. Understanding these connections will help in the diagnosis and treatment of cancer and other diseases.
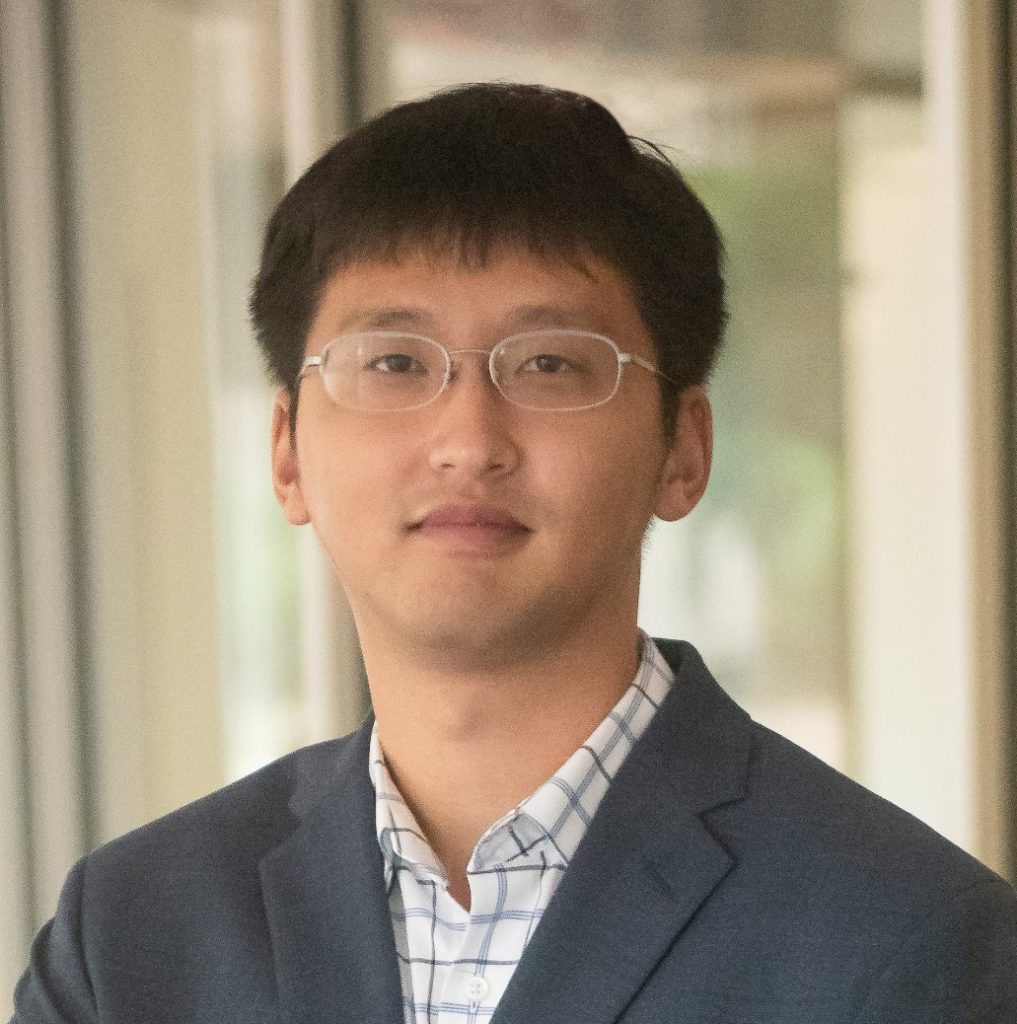
Yang Ni, Ph.D., an assistant professor in the College of Arts and Sciences Department of Statistics, will be the principal investigator for the effort. The project, which aims to create a toolset for interpreting and correlating novel genetic information, is titled “Bayesian differential causal network and clustering methods for single-cell data.”
Co-investigators for the project will be Robert Chapkin, Ph.D, Distinguished Professor and Allen Endowed Chair in the College of Agriculture and Life Sciences Department of Nutrition and Department of Biochemistry and Biophysics, and James Cai, Ph.D., associate professor in the School of Veterinary Medicine and Biomedical Sciences Department of Veterinary Integrative Biosciences.
The project aims to advance single-cell data science, the study of how genes and gene expression differ among individual cells in one organism. Because cancer arises from genetic abnormalities in individual cells, scientists believe that single-cell data science will reveal medically important information.
This NIH award is tied to a pending grant to the Cancer Prevention and Research Institute of Texas, CPRIT, to assess gene-environment-lifestyle interactions in cancer. Chapkin is spearheading that effort in collaboration with Ken Ramos, M.D., Ph.D., executive director at Texas A&M’s Institute of Biosciences and Technology.
Ramos and Chapkin have also submitted a $6 million grant titled “Gene-environment-lifestyle interactions in cancer” to the CPRIT to create a new regional center of excellence in cancer research.
Chapkin said the NIH grant’s goals and personnel will complement the establishment of a single-cell data science core at Texas A&M that will serve as a shared-resource facility.
Purpose and goals of single-cell technology
The emergence of new technologies such as single-cell RNA sequencing and spatial profiling has brought about many methods to study gene regulation and cell differentiation in the single cells of multicellular organisms.
Ni will lead the effort, along with students and postdocs, to develop and implement new statistical methods for discovering causal gene regulation and molecular cell types with single-cell RNA-sequencing data.
“We will also work closely with Drs. Chapkin and Cai to solve real-world biological and biomedical problems,” he said.
“New methods are required to compare molecular differences at the single-cell level, so we can translate the knowledge advanced by single-cell RNA sequencing and spatial mapping to improve disease diagnosis, treatment and prevention,” Ni said.
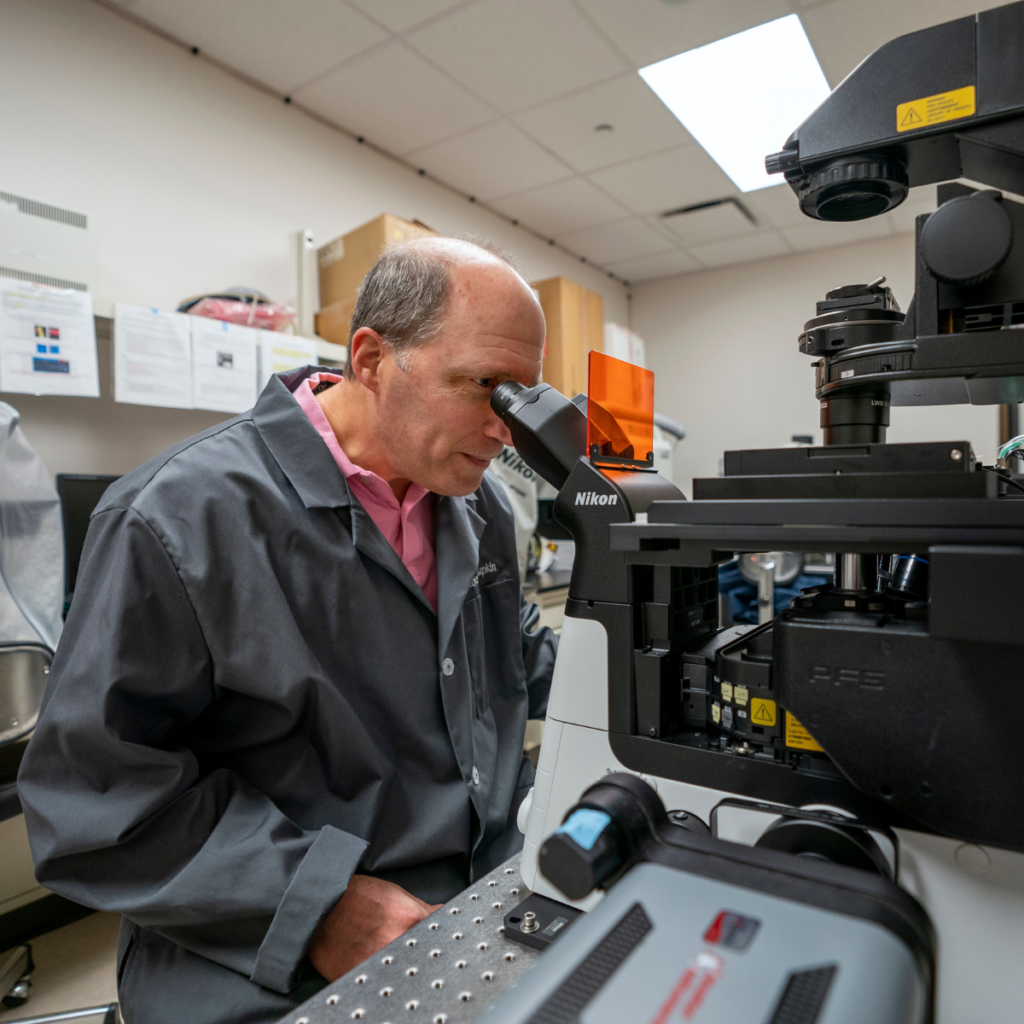
Single-cell technology and high-throughput sequencing have enabled entirely new realms of biology, such as “deep phenotyping” and personalized medicine.
“In these applications, big data provide insights into cures for various diseases, including cancer,” Chapkin said.
Single-cell data allows for the detection of cell-to-cell interactions, gene networks and the spatial organization of gene expression in tissue samples.
“There is a consensus that single-cell multiomics data, in which data sets of different groups are combined during analysis, and spatial information will be driving next-generation solutions in oncology,” Chapkin said.
He said this type of single-cell multiomic research, is highly innovative.
“It will enable the characterization of previously unapproachable clinical phenomena, such as ’deep landscapes’ of cancer heterogeneity that will reveal more about the dynamics of the tumor microenvironment,” Chapkin said.
Ni said a long-term goal of the project is to develop novel statistical methods for generating and evaluating new hypotheses about complex cellular processes across disparate sample groups, such as disease subtypes and treatment groups.
Specifically, Ni said the team will work to design and validate Bayesian network and clustering models. The novel statistical models and related analytical tools will help accurately describe changes in gene regulation and cell differentiation in response to experimental interventions at the single-cell level under different experimental conditions.
“Without such tools, mechanistically understanding gene regulatory activities and cell differentiation will likely remain difficult,” he said. “And the proposed methods would be widely applicable to a range of data generated under different experimental conditions, disease subtypes and treatment groups.”
Single-cell modeling and connecting
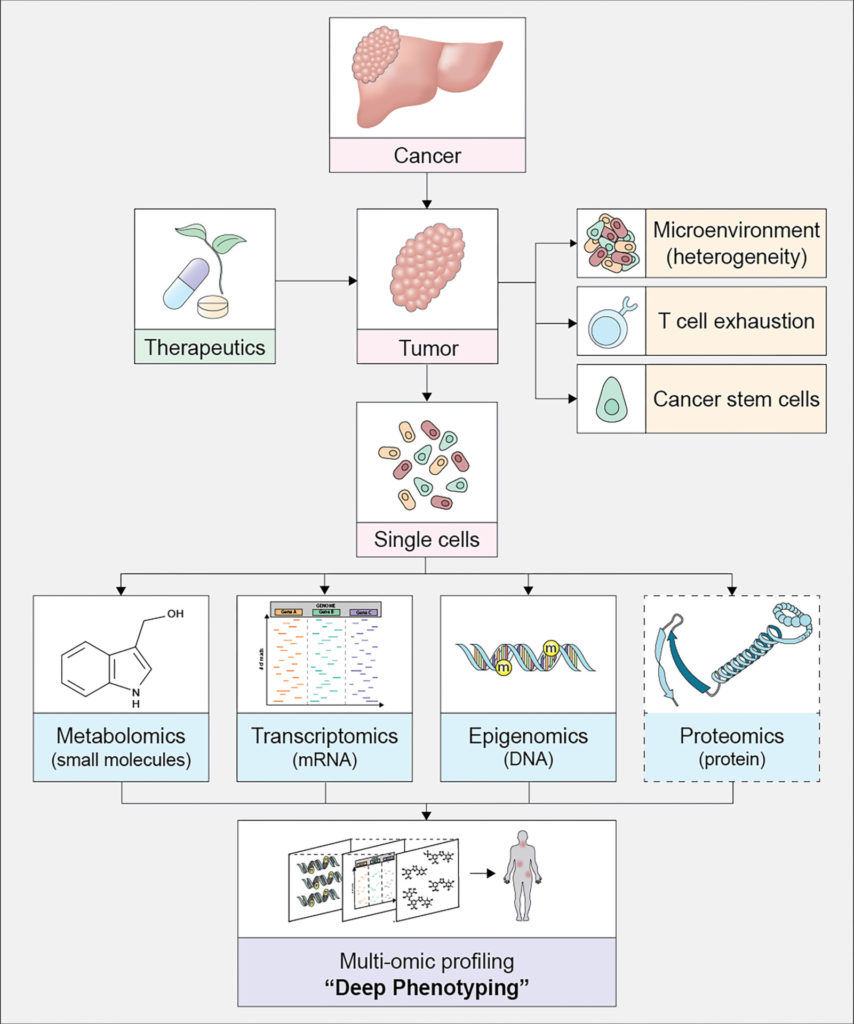
A Bayesian network model governs the probability and causal rules of a set of variables. An example would be a Bayesian network representing the probable relationships between diseases and their symptoms.
“In a Bayesian network model, first you identify main variables in the problem you want to solve,” explained Ni. “Then you specify or learn the structure of the network, which is the causal relationships between all the variables identified. After that, you determine the probability rules governing the relationships between those variables.”
Cai is also an affiliated faculty member in the College of Engineering Department of Electrical and Computer Engineering, and the Cai lab members develop analytical frameworks to study single-cell genomics data gathered from various types of cells.
In this project, he will play a bridging and coordinating role by connecting statisticians with molecular biologists to create an interface between human genetics, computational statistics and data science.
Sharing the results
Ni will lead the effort to disseminate the project’s results more broadly through open-source software, conference presentation, graduate and undergraduate education and mentoring, and hosting interdisciplinary workshops and symposia.
“We’re arranging for these workshops to provide travel stipends to help underrepresented students in STEM and encourage their participation,” he noted.
To help prepare next-generation researchers, statisticians and data scientists and improve public statistical literacy and engagement, Ni will also host data science competitions designed to engage undergraduate students.
“I also plan to develop a new graduate course on causal networks, and supervise the research of postdoctoral, graduate and undergraduate researchers,” he said.